Hi,
You might want to look into BERT and GPT-3, these are Transformer based architectures.
Bert uses only the Encoder part, whereas GPT-3 uses only the Decoder part.
Both of them have the ability to predict tokens, but in different ways.
For simplicity I will show you some examples with a library that has these models pretrained called HuggingFace. (Even this pytorch documentation for transformers refers to this library. To install simply run pip install transformers
)
One of the use cases for BERT is to predict what the [MASK] token should be, taking the whole sentence into consideration. link
from transformers import pipeline
unmasker = pipeline('fill-mask', model='bert-base-cased')
unmasker("Paris is the [MASK] of France.")
# Ouput:
[{'score': 0.9861817359924316,
'sequence': 'Paris is the capital of France.',
'token': 2364,
'token_str': 'capital'},
{'score': 0.0037214006297290325,
'sequence': 'Paris is the center of France.',
'token': 2057,
'token_str': 'center'},
{'score': 0.003259770106524229,
'sequence': 'Paris is the Capital of France.',
'token': 6299,
'token_str': 'Capital'},
{'score': 0.0021571300458163023,
'sequence': 'Paris is the centre of France.',
'token': 2642,
'token_str': 'centre'},
{'score': 0.0009026133338920772,
'sequence': 'Paris is the city of France.',
'token': 1331,
'token_str': 'city'}]
For the GPT we can look at the use case to predict the next words, given a starting point. Here I am actually using GPT-2, but it is the same concept. link
from transformers import pipeline, set_seed
generator = pipeline('text-generation', model='gpt2')
set_seed(42)
generator("Hello, I'm learning about machine learning,", max_length=30, num_return_sequences=5)
# Output:
[{'generated_text': "Hello, I'm learning about machine learning, I'm learning from it. In fact, this summer I think the field of machine learning may be really"},
{'generated_text': "Hello, I'm learning about machine learning, which is, again, why I'm posting a bunch of tutorials about it. This isn't a bad"},
{'generated_text': 'Hello, I\'m learning about machine learning, but I don\'t get much of a connection anymore with the new machine learning tools I\'m getting into."'},
{'generated_text': "Hello, I'm learning about machine learning, a more general term here.\n\nThis article will show you how to make a machine learning framework for"},
{'generated_text': "Hello, I'm learning about machine learning, but I like my work because I like to teach. I need to give myself a good foundation so that"}]
This being said, you can look into fine-tuning these models, or other variations of them (there are a lot).
But if you want to train them from scratch, they are going to need A LOT of data and processing resources. (e.g. BERT used the entire English wikipedia to train)
Regarding this:
You can plot a heatmap of your output to see what is actually happening.
import math
import torch
import seaborn as sns
import matplotlib.pyplot as plt
words = 20
T = torch.nn.Transformer(num_encoder_layers=0)
seq = torch.rand(words, 512)
out = T(seq, seq)
with torch.no_grad():
f,(ax1,ax2,ax3) = plt.subplots(1,3,figsize=(11,5), gridspec_kw={'width_ratios':[.5,.5,0.05]})
map1 = seq @ seq.T
sns.heatmap(map1.numpy(),cmap="YlGnBu",cbar=False,ax=ax1)
map2 = seq @ out.T
sns.heatmap(map2.numpy(),cmap="YlGnBu",ax=ax2,cbar_ax=ax3)
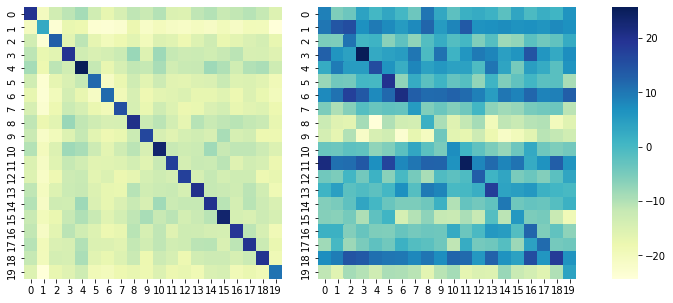
This would be an example for a sentence with 20 “words”. On the left you see the confusion matrix for the input values against the input values. Since they are the same, we get a strong diagonal, whereas all other values seem to have little to no relation. On the right we have the input values against the output values. Here the diagonal is also strong, however all other have undergone transformations that would place word with similar semantic context closer to each other due to the self attention mechanism (as well as the positional embedding).
So the answer would be, yes, they are attending to itself. And you can play around with masking the source or the target.
Hope this helps 
Let me know if something is not clear or you need more help.
(Bonus: BertViz is a very cool library that can help you visualize the attention heads of BERT and how the words affect each other.)