I actually thick that the convLSTM causes the problem. Even, when I have a single convLSTM as my model, in the first iteration, only the outer layers have gradient and the rest are zero. See figure1.
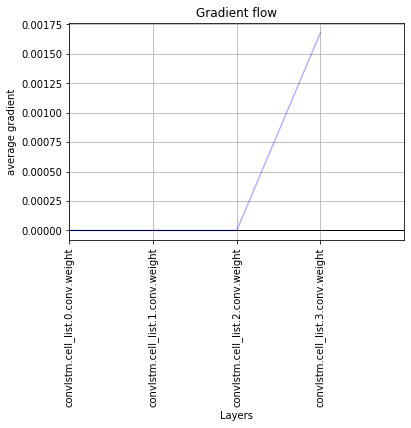
If I keep doing the calculation, again, the gradients all go to zero! See figure 2.
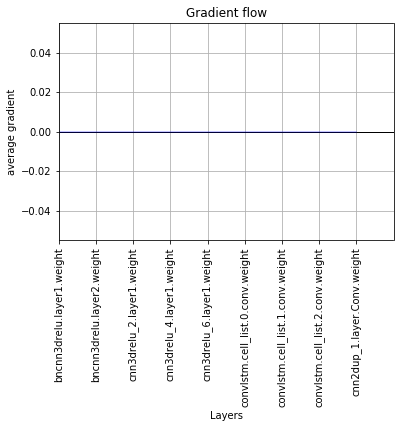
I did not find any built-in function for convLSTM and I’m using the following code to have them. I do appreciate your comments if you have better code, or tell me if there is any problem in the code.
class ConvLSTMCell(nn.Module):
def __init__(self, input_size, input_dim, hidden_dim, kernel_size, bias, device):
"""
Initialize ConvLSTM cell.
Parameters
----------
input_size: (int, int)
Height and width of input tensor as (height, width).
input_dim: int
Number of channels of input tensor.
hidden_dim: int
Number of channels of hidden state.
kernel_size: (int, int)
Size of the convolutional kernel.
bias: bool
Whether or not to add the bias.
"""
super(ConvLSTMCell, self).__init__()
self.height, self.width = input_size
self.input_dim = input_dim
self.hidden_dim = hidden_dim
self.kernel_size = kernel_size
self.padding = kernel_size[0] // 2, kernel_size[1] // 2
self.bias = bias
self.conv = nn.Conv2d(in_channels=self.input_dim + self.hidden_dim,
out_channels=4 * self.hidden_dim,
kernel_size=self.kernel_size,
padding=self.padding,
bias=self.bias)
self.device = device
def forward(self, input_tensor, cur_state):
h_cur, c_cur = cur_state
combined = torch.cat([input_tensor, h_cur], dim=1).to(self.device) # concatenate along channel axis
combined_conv = self.conv(combined).to(self.device)
cc_i, cc_f, cc_o, cc_g = torch.split(combined_conv, self.hidden_dim, dim=1)
i = torch.sigmoid(cc_i)
f = torch.sigmoid(cc_f)
o = torch.sigmoid(cc_o)
g = torch.tanh(cc_g)
c_next = f * c_cur + i * g
h_next = o * torch.tanh(c_next)
return h_next, c_next
def init_hidden(self, batch_size):
return (Variable(torch.zeros(batch_size, self.hidden_dim, self.height, self.width), requires_grad = True).to(self.device),
Variable(torch.zeros(batch_size, self.hidden_dim, self.height, self.width), requires_grad = True).to(self.device))
class ConvLSTM(nn.Module):
def __init__(self, input_size, input_channels, hidden_dim, kernel_size, num_layers,
target=[[0,0]], bias=True, return_all_layers=False, device='cuda:0'):
super(ConvLSTM, self).__init__()
self._check_kernel_size_consistency(kernel_size)
self.device = device
self.target = target
# Make sure that both `kernel_size` and `hidden_dim` are lists having len == num_layers
kernel_size = self._extend_for_multilayer(kernel_size, num_layers)
hidden_dim = self._extend_for_multilayer(hidden_dim, num_layers)
if not len(kernel_size) == len(hidden_dim) == num_layers:
raise ValueError('Inconsistent list length.')
self.height, self.width = input_size
self.input_dim = input_channels
self.hidden_dim = hidden_dim
self.kernel_size = kernel_size
self.num_layers = num_layers
self.bias = bias
self.return_all_layers = return_all_layers
cell_list = []
for i in range(0, self.num_layers):
cur_input_dim = self.input_dim if i == 0 else self.hidden_dim[i-1]
cell_list.append(ConvLSTMCell(input_size=(self.height, self.width),
input_dim=cur_input_dim,
hidden_dim=self.hidden_dim[i],
kernel_size=self.kernel_size[i],
bias=self.bias,
device = self.device))
self.cell_list = nn.ModuleList(cell_list)
def forward(self, input_tensor, hidden_state=None):
# Implement stateful ConvLSTM
if hidden_state is not None:
raise NotImplementedError()
else:
hidden_state = self._init_hidden(batch_size=input_tensor.size(0))
layer_output_list = []
last_state_list = []
out = torch.tensor([]).to(self.device)
seq_len = input_tensor.size(2)
cur_layer_input = input_tensor
for layer_idx in range(self.num_layers):
h, c = hidden_state[layer_idx]
output_inner = []
for t in range(seq_len):
h, c = self.cell_list[layer_idx](input_tensor=cur_layer_input[:, :, t, :, :],
cur_state=[h, c])
output_inner.append(h)
layer_output = torch.stack(output_inner, dim=2)
cur_layer_input = layer_output
layer_output_list.append(layer_output)
last_state_list.append([h, c])
if [t, layer_idx] in self.target:
out = torch.cat([out , h], 1)
return out
def _init_hidden(self, batch_size):
init_states = []
for i in range(self.num_layers):
init_states.append(self.cell_list[i].init_hidden(batch_size))
return init_states
@staticmethod
def _check_kernel_size_consistency(kernel_size):
if not (isinstance(kernel_size, tuple) or
(isinstance(kernel_size, list) and all([isinstance(elem, tuple) for elem in kernel_size]))):
raise ValueError('`kernel_size` must be tuple or list of tuples')
@staticmethod
def _extend_for_multilayer(param, num_layers):
if not isinstance(param, list):
param = [param] * num_layers
return param